Journal of Artificial Intelligence Research & Advances (JoAIRA)
ISSN: 2395-6720
Click here for complete Editorial Board
Scientific Journal Impact Factor (SJIF): 7.464
Journal of Artificial Intelligence Research & Advances (JoAIRA) is a journal focused towards the rapid publication of fundamental research papers on all areas of Artificial Intelligence Research & Advances. It's a triannual journal, started in 2014.
Journal DOI no.: 10.37591/JoAIRA
Focus and Scope Cover
- Artificial intelligence and Philosophy
- Case based reasoning
- Heuristic searching
- Intelligent robotics
- Planning and theories of action
- Cognitive aspects of AI
- Robotics
- Soft computing theory and applications
- Evolutionary inspired computing
- Pervasive computing and ambient intelligence
- Intelligent Web
- Bioinformatics
- Neuroinformatics
- Computing and the mind
- Cognitive science
- Non-classical computing and novel computing models
- DNA computing and quantum computing
- Natural computing
Readership: Graduate, Postgraduate, Research Scholar, Faculties, Institutions, and in IT Companies.
Indexing: The Journal is index in DRJI, Google Scholar
Submission of Paper:
All contributions to the journal are rigorously refereed and are selected on the basis of quality and originality of the work. The journal publishes the most significant new research papers or any other original contribution in the form of reviews and reports on new concepts in all areas pertaining to its scope and research being done in the world, thus ensuring its scientific priority and significance.
Manuscripts are invited from academicians, students, research scholars and faculties for publication consideration.
Papers are accepted for editorial consideration through email [email protected] or [email protected]
Abbreviation: JoAIRA
Frequency: Three issues per year
Publisher: STM Journals A division of: Consortium eLearning Network Private Ltd
Address: A-118, 1st Floor, Sector-63, Noida, Uttar Pradesh-201301, India
Phone no.: 0120-4746-214/ Email: [email protected]
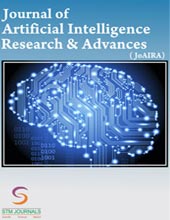
Publisher: STM Journals, an imprint of CELNET (Consortium e-Learning Network Pvt. Ltd.)
Address: A-118, 1st Floor, Sector-63, Noida, Uttar Pradesh-201301, India
Phone no.: 0120-4746-214/ Email: [email protected]
This site has been shifted to https://stmcomputers.stmjournals.com/